The third post from my Just Two Things newsletter on Artificial Intelligence.
The notion that algorithms are a source of social disadvantage because they encode existing biases into technology systems is rapidly crossing over into the political mainstream.
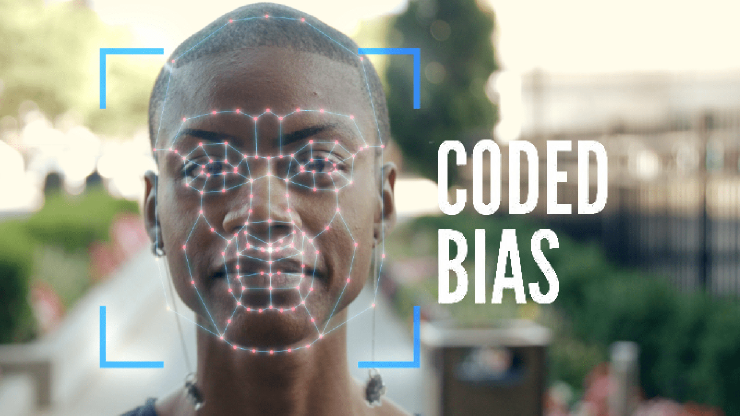
The documentary Coded Bias, now on Netflix, is more proof of this. The film has been made by activist director Shalina Kantayya. I haven’t seen it yet, but the South African magazine Daily Maverick has a recent review article.
The film is bookended by Joy Buolamwini, who is now known as the founder of the Algorithmic Justice League. Her journey into this world started at MIT:
she was making an art-science project that could detect faces in a mirror and project other faces and images over them. But the detection software seemed unable to detect black faces. Buolamwini’s curiosity piqued, she went about researching the data set images used to teach face detection algorithms to see, and what she discovered was that there were significantly fewer black faces in these data sets.
Of course there were. But when these types of software are deployed by police forces, the outcome is the misidentification of black people as suspects: “At one point we see four undercover London policemen stop and question a 14-year-old black boy in school uniform because his face matched with their criminal database.”
The racist applications of algorithms are well know now, I think, but there’s been less discussion of how they act to reinforce the status quo.
Imagine an algorithm that is purportedly designed to evaluate the highest performing demographic in a company to determine what kind of person to hire. It would only be able to “learn” from employees who have already worked at that company… This is exactly what happened at Amazon when they tried to use an algorithm to assess resumes of potential employees. Because the company was dominated by men, the highest achieving employees were – you guessed it – men. So the algorithm learned to reject any resume from a female applicant.
But then again: the tech campaigner Cathy O’Neill defines an algorithm as “using historical information to make a prediction about the future.” The problem is built into the structure.
One of the features of this whole area is that while only 14% of AI researchers are women, they are almost the only voices heard making these critiques. John Naughton noted this point in a recent Observer/Guardian column. It’s not chance that the two people who left Google recently over an article on AI ethics are both women. Almost all the interviewees in Coded Bias are women.
But then again: as Sinclair Lewis once said, “It is difficult to get a man to understand something, when his salary depends upon his not understanding it.” Even the apparently reformed tech bros who pop up in programmes about the issues caused by the growth of pervasive digital systems seems to have problems seeing this as a systemic issue.
It is striking how effective these women critics have been. In particular they have managed to pull the issue into the public sphere, so that figures who’re not associated with technology research have joined the fray. One more piece of evidence of this: Daron Acemoglu, better known for his research on states, power, and prosperity, has a long, long piece in Boston Review on the need for policy responses to shape the application of AI:
The direction of AI development is not preordained. It can be altered to increase human productivity, create jobs and shared prosperity, and protect and bolster democratic freedoms—if we modify our approach. In order to redirect AI research toward a more productive path, we need to look at AI funding and regulation, the norms and priorities of AI researchers, and the societal oversight guiding these technologies and their applications.
The trailer for Coded Bias is here.