One of the dirty secrets of artificial intelligence and the Large Language Models that sit behind them is that behind the technology there is always a worker, often low paid, often in a remote global location, often recruited through a website, almost always working casually, on piece-rates.
Kate Crawford and Vladan Joler touched on this a couple of years ago, when they dissected the entire system of Amazon’s Alexa, which Peter Curry wrote about on Just Two Things. Now a long, long article in New York magazine—written in collaboration with The Verge—has gone into the world of the workers who train large language models.
All I’m going to do here is to skate across the surface of it, and if you’re interested in the way tech actually works (rather than the way that Silicon Valley says it works) it’s definitely worth some of your time. You get the flavour, though, early on:
Much of the public response to language models like OpenAI’s ChatGPT has focused on all the jobs they appear poised to automate. But behind even the most impressive AI system are people — huge numbers of people labeling data to train it and clarifying data when it gets confused.
Confusing work
The article starts in Kenya: the money’s better than the alternatives, but the work itself is confusing. You are basically a human teaching a device how a human would respond to something, but you don’t usually get a sense of the whole:
Labeling objects for self-driving cars was obvious, but what about categorizing whether snippets of distorted dialogue were spoken by a robot or a human? Uploading photos of yourself staring into a webcam with a blank expression, then with a grin, then wearing a motorcycle helmet? Each project was such a small component of some larger process.
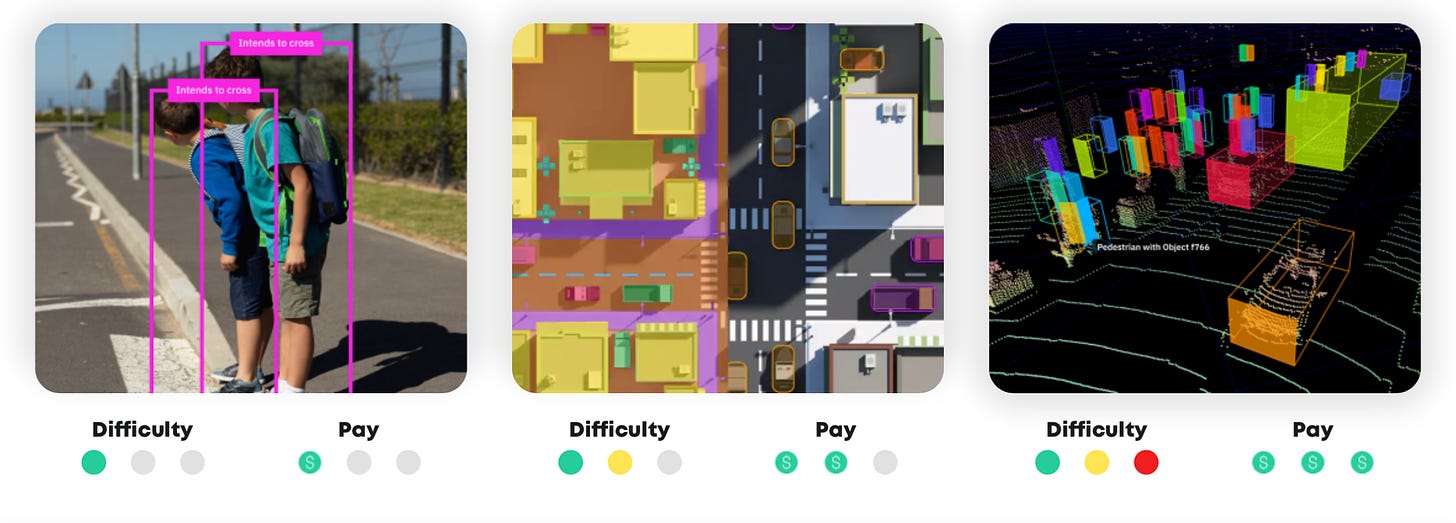
Dzieza describes these as the inverse of David Graeber’s idea of ‘bullshit jobs’, by which Graeber meant work that had no meaning or purpose:
These AI jobs are their bizarro twin: work that people want to automate, and often think is already automated, yet still requires a human stand-in. The jobs have a purpose; it’s just that workers often have no idea what it is.
Labelling the data
Labelling data is at the heart of Large Language Models, and the theory used to be that after a while you’d have enough labels on enough data, and the program would be able to work out the rest for itself. But it turns out, in effect, that there’s never enough data and it always needs labelling.
You collect as much labeled data as you can get as cheaply as possible to train your model, and if it works, at least in theory, you no longer need the annotators. But annotation is never really finished. Machine-learning systems are what researchers call “brittle,” prone to fail when encountering something that isn’t well represented in their training data. These failures, called “edge cases,” can have serious consequences.
Consequences
Consequences like killing a woman wheeling a bicycle across a road, as an Uber self-driving car did in 2018. The program had been trained on pedestrians, and trained on cyclists, but didn’t recognise them in combination. There are always edge cases: using web-recruited annotators, and paying them by the task, is a way to make the labour pool bigger to get through more edge cases. Dzieza set out to talk to these annotators:
(W)hile many of them were training cutting-edge chatbots, just as many were doing the mundane manual labor required to keep AI running. There are people classifying the emotional content of TikTok videos, new variants of email spam, and the precise sexual provocativeness of online ads. Others are looking at credit-card transactions and figuring out what sort of purchase they relate to or checking e-commerce recommendations and deciding whether that shirt is really something you might like after buying that other shirt. Humans are correcting customer-service chatbots, listening to Alexa requests, and categorizing the emotions of people on video calls.
Simplifying reality
The work is almost all outsourced, and the workers who do it are required to sign strict non-disclosure agreements. Most of Dzieza’s annotators requested anonymity for his article. It’s hard to know how many people are in this supply chain: the article quotes a Google research report that says “millions”, but that this could grow to “billions”. So nobody has a clue, in other words.
He also signs up for the work himself, in an entertaining section (he struggles). The instructions to the annotators have to be literal and deal with all options. The reason for that, of course, is that a Large Language Model has no context: it only has a label that it has learnt:
The act of simplifying reality for a machine results in a great deal of complexity for the human. Instruction writers must come up with rules that will get humans to categorize the world with perfect consistency. To do so, they often create categories no human would use. A human asked to tag all the shirts in a photo probably wouldn’t tag the reflection of a shirt in a mirror because they would know it is a reflection and not real. But to the AI, which has no understanding of the world, it’s all just pixels and the two are perfectly identical.
Thinking like a robot
And so the challenge of an annotator ends up in a strange doppelganger world where a human has to think like a robot, so that a machine can pretend to be a human:
It’s a strange mental space to inhabit, doing your best to follow nonsensical but rigorous rules, like taking a standardized test while on hallucinogens. Annotators invariably end up confronted with confounding questions like, Is that a red shirt with white stripes or a white shirt with red stripes? Is a wicker bowl a “decorative bowl” if it’s full of apples? What color is leopard print?
Some of the most interesting material in the article is about how the annotators club together to manage the vagaries of the system, especially in places like Kenya. They also help people to learn which tasks to stay away from (the ones that are both poorly paid and complex are terrible) and share tips on doing the work effectively.
Training chatbots
Some of the better paid work involves training chatbots, and that tends to be closer to home, in the United States. ‘Anna’ is paid to talk to ChatGPT for seven hours a day, and improve its responses. She enjoys the work:
She has discussed science-fiction novels, mathematical paradoxes, children’s riddles, and TV shows. Sometimes the bot’s responses make her laugh… Each time Anna prompts Sparrow, it delivers two responses and she picks the best one, thereby creating something called “human-feedback data.”
Human factors
What’s going on here? It’s the same story: it’s the humans in the training loop that makes ChatGPT appear to be, well, human:
ChatGPT seems so human because it was trained by an AI that was mimicking humans who were rating an AI that was mimicking humans who were pretending to be a better version of an AI that was trained on human writing.
Some technologists think that the machines will get smart enough not to need this “reinforcement learning from human feedback” (RLHF). Reading the article, this doesn’t seem likely. It seems more likely that as the models become more complex and more sophisticated, the demands we make of them will increase, and so the edge cases will also become more complex. And so the demands made of the humans in the learning cycle will also become more complex.
—-
A version of this article is also published on my Just Two Things Newsletter.